Blog
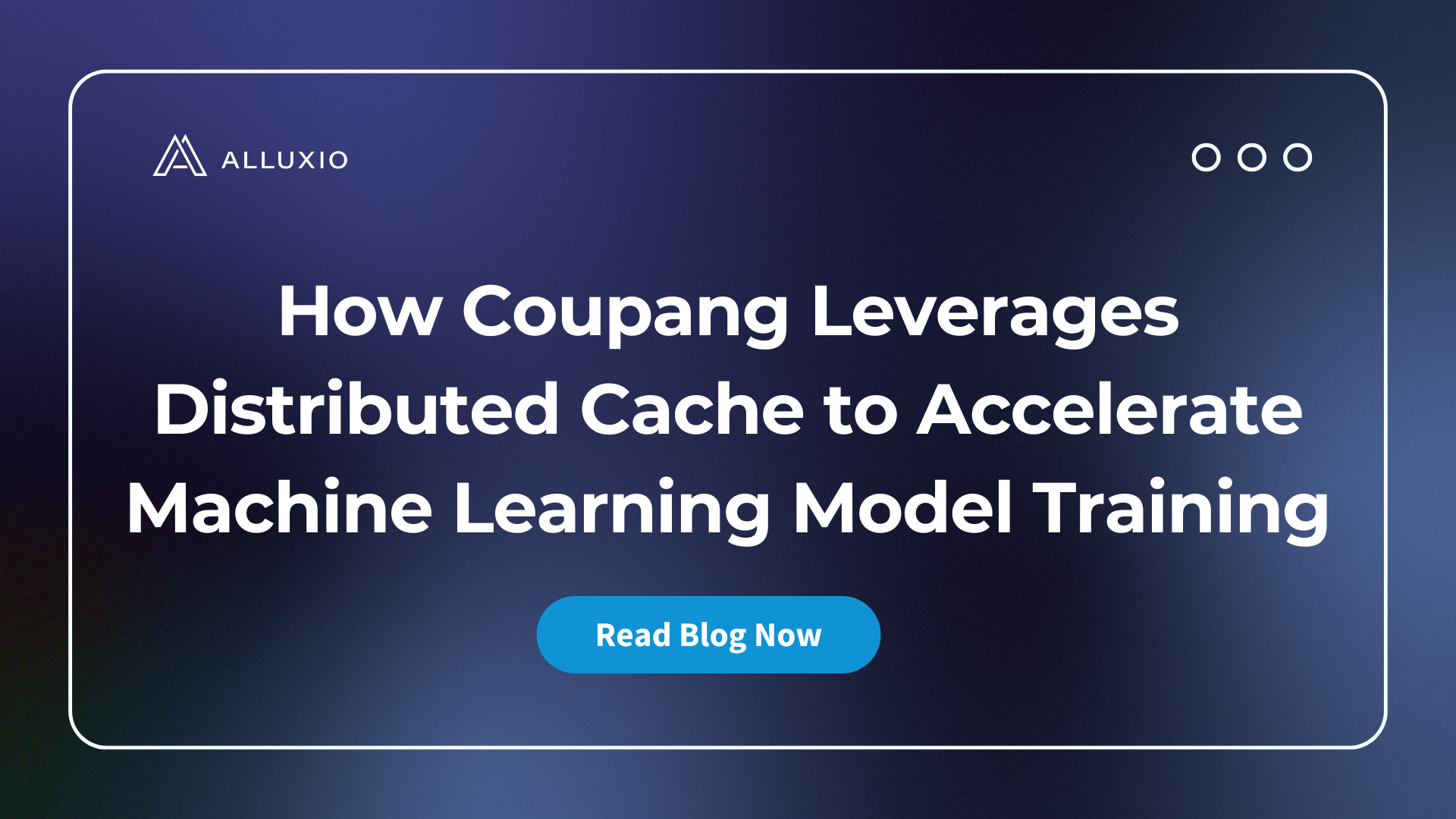
Coupang, a Fortune 200 technology company, manages a multi-cluster GPU architecture for their AI/ML model training. This architecture introduced significant challenges, including:
- Time-consuming data preparation and data copy/movement
- Difficulty utilizing GPU resources efficiently
- High and growing storage costs
- Excessive operational overhead maintaining storage for localized data silos
To resolve these challenges, Coupang’s AI platform team implemented a distributed caching system that automatically retrieves training data from their central data lake, improves data loading performance, unifies access paths for model developers, automates data lifecycle management, and extends easily across Kubernetes environments. The new distributed caching architecture has improved model training speed, reduced storage costs, increased GPU utilization across clusters, lowered operational overhead, enabled training workload portability, and delivered 40% better I/O performance compared to parallel file systems.
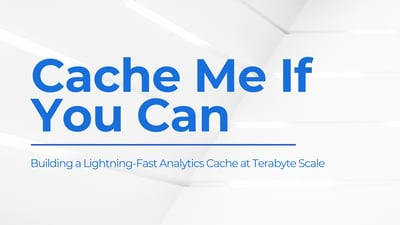
Suresh Kumar Veerapathiran and Anudeep Kumar, engineering leaders at Uptycs, recently shared their experience of evolving their data platform and analytics architecture to power analytics through a generative AI interface. In their post on Medium titled Cache Me If You Can: Building a Lightning-Fast Analytics Cache at Terabyte Scale, Veerapathiran and Kumar provide detailed insights into the challenges they faced (and how they solved them) scaling their analytics solution that collects and reports on terabytes of telemetry data per day as part of Uptycs Cloud-Native Application Protection Platform (CNAPP) solutions.
.png)
.jpeg)
Today, I’m thrilled to announce the GA of Alluxio 2.0.0, Alluxio’s biggest release to date (see our Release Notes & Release Blog) with over 900 commits.
.jpeg)
This article aims to provide a different approach to help connect and make distributed files systems like HDFS or cloud storage systems look like a local file system to data processing frameworks: the Alluxio POSIX API. To explain the approach better, we used the TensorFlow + Alluxio + AWS S3 stack as an example.

Alluxio is a proud sponsor and exhibitor at the Presto Summit in San Francisco. If you missed the conference, don’t worry we’ve got you covered!
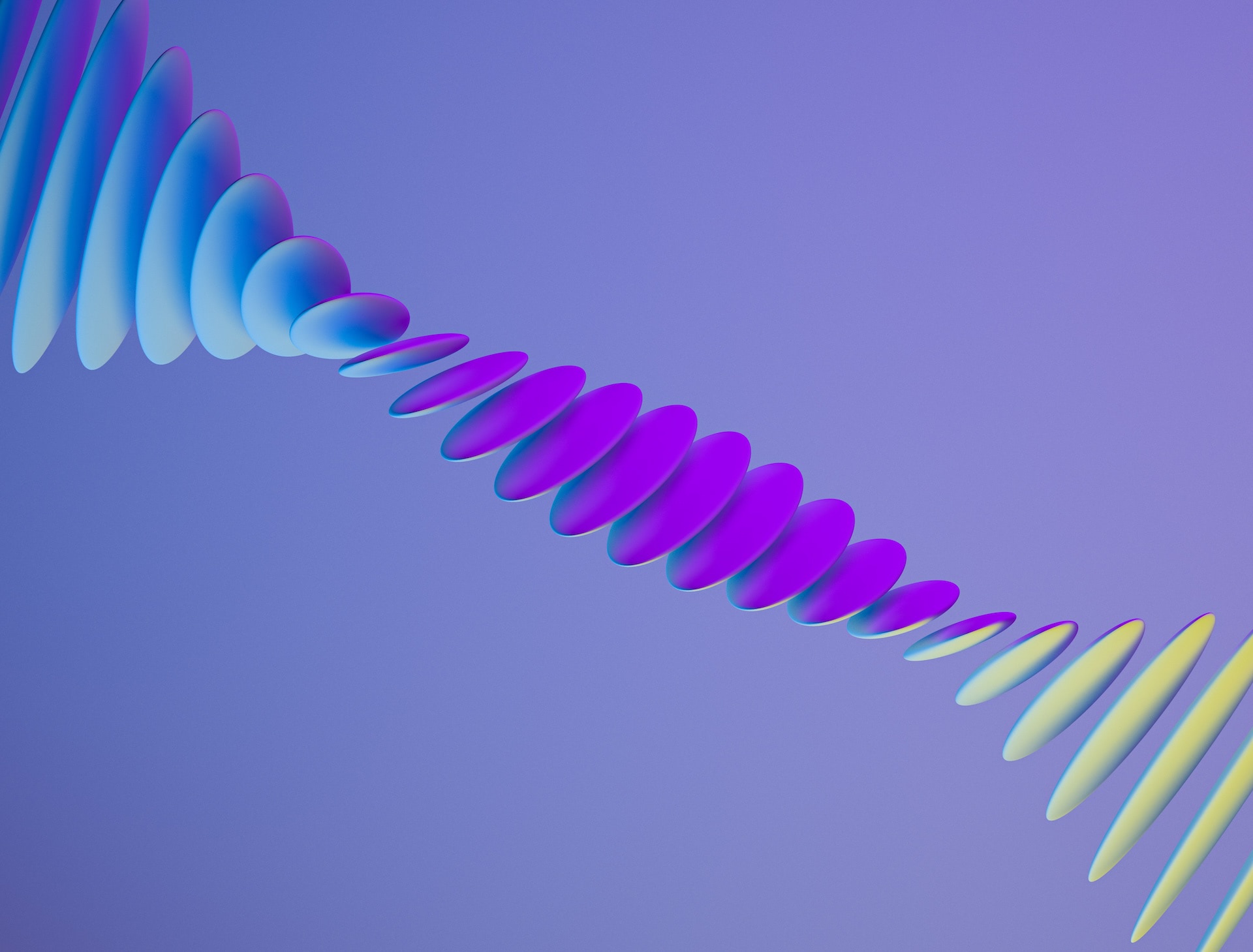
As the data ecosystem becomes massively complex and more and more disaggregated, data analysts and end users have trouble adapting and working with hybrid environments. The proliferation of compute applications along with storage mediums leads to a hybrid model that we are just not accustomed to. With this disaggregated system data engineers now come across a multitude of problems that they must overcome in order to get meaningful insights.

Over the years of working in the big data and machine learning space, we frequently hear from data engineers that the biggest obstacle to extracting value from data is being able to access the data efficiently. Data silos, isolated islands of data, are often viewed by data engineers as the key culprit or public enemy №1. There have been many attempts to do away with data silos, but those attempts themselves have resulted in yet another data silo, with data lakes being one such example. Rather than attempting to eliminate data silos, we believe the right approach is to embrace them.
.jpeg)
Cloud has changed the dynamics of data engineering as well as the behavior of data engineers in many ways. This is primarily because a data engineer on premise only dealt with databases and some parts of the hadoop stack. In the cloud, things are a bit different. Data engineers suddenly need to think different and broader. Instead of being purely focused on data infrastructure, you are now almost a full stack engineer (leaving out the final end application perhaps). Compute, containers, storage, data movement, performance, network — skills are increasing needed across the broader stack. Here are some design concept and data stack elements to keep in mind.

Announcing the OEM partnership with Alluxio and Starburst Data, the company behind Presto, the fastest growing SQL query engine in a disaggregated world.
.jpeg)
Traditionally, if you want to run a single Spark job on EMR, you might follow the steps: launching a cluster, running the job which reads data from storage layer like S3, performing transformations within RDD/Dataframe/Dataset, finally, sending the result back to S3. You end up having something like this. If we add more Spark jobs across multiple clusters, you could have something like this.
.jpeg)
This article walks through the journey of a startup HashData in Beijing to build a cloud-native high-performance MPP shared-everything architecture leveraging object storage as the data persistence layer and Alluxio as a data orchestration layer in the cloud. we will illustrate how HDW leverages Alluxio as the data orchestration layer to eliminate the performance penalty introduced by object storage while benefiting from its scalability and cost-effectiveness.
.jpeg)
Discontinuity in big data infrastructure drives storage disaggregation, especially in companies experiencing dramatic data growth after pivoting to AI and analytics. This data growth challenge makes disaggregating storage from compute attractive because the company can scale their storage capacity to match their data growth, independent of compute. This decoupled mode allows the separation of compute and storage, enabling users to rightsize hardware for each layer. Users can buy high-end CPU and memory configurations for the compute nodes, and storage nodes can be optimized for capacity. This whitepaper is a continuation of Unlock Big Data Analytics Efficiency with Compute and Storage Disaggregation on Intel® Platforms
.jpeg)
This is a guest blog by Jowanza Joseph with an original blog source. It is about how he used Alluxio to reduce p99 and p50 query latencies and optimized the overall platform costs for a distributed querying application. Jowanza walks through the product and architecture decisions that lead to our final architecture, discuss the tradeoffs, share some statistics on the improvements, and discuss future improvements to the system.
.jpeg)
Alluxio provides a unified namespace where you can mount multiple different storage systems and access them through the same API. To serve the file system requests to operate on all the files and directories in this namespace, Alluxio masters must handle the file system metadata at a scale of all mounted systems combined. We are writing several engineering blogs describing the design and implementation of Alluxio master to address this scalability challenge. This is the first article focusing on metadata storage and service, particularly how to use RocksDB as an embedded persistent key-value store to encode and store the file system inode tree with high performance.