ABOUT THE COMPANY
This Alluxio Enterprise AI customer is a publicly traded e-commerce company with over 50,000 employees and $20+ billion in annual revenue. The company sells apparel, electronics, toys, food, and other products marketed.
CHALLENGE
The e-commerce company builds and trains AI/ML models to enhance and customize product search results and product recommendations for their 100+ million customers. Their training data, stored in AWS S3 in the “S” region, has grown to 100’s of petabytes. The AI/ML training workloads were distributed across multiple AWS regions as well as in an on-premises data center.
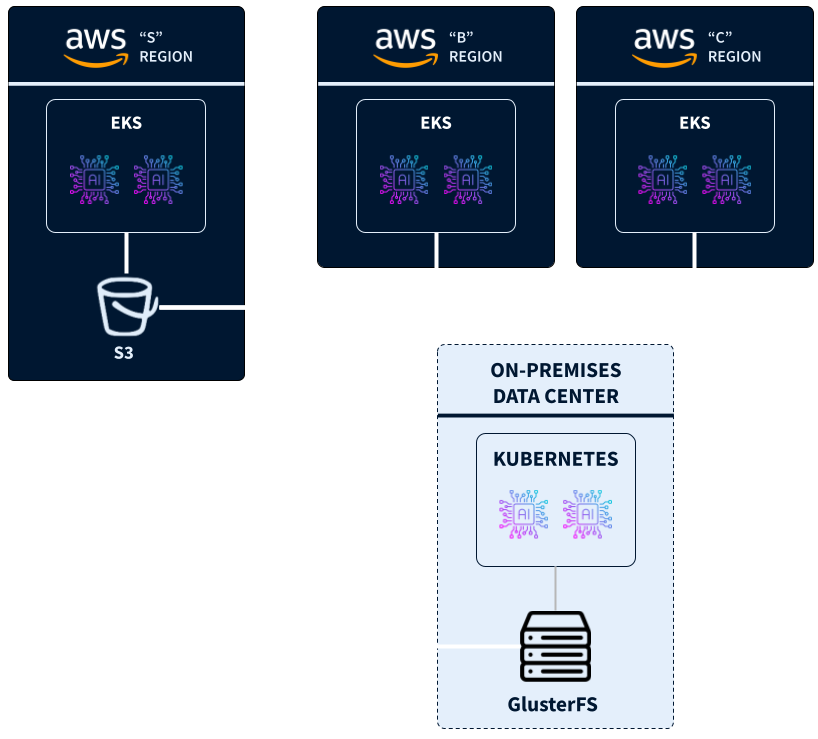
AI/ML training workloads running on AWS accessed training data directly from S3 in the “S” Region. Each training workload running in their on-premises data center downloaded training data from S3 and stored it on networked attached storage with GlusterFS.
With this strategy, the company suffered from storage and network bandwidth constraints, causing AI/ML training workloads to be slow and unstable. Additionally, The e-commerce company faced:
- High AWS S3 API and egress costs
- Low GPU utilization during training jobs
- High cost and operational complexity of managing GlusterFS and associated hardware
SOLUTION
After evaluating several high-performance storage solutions, the company selected Alluxio AI Enterprise to solve these business-critical challenges due to Alluxio’s innovative distributed caching technology.
Alluxio Distributed Cache clusters are deployed in each AWS region as well as their on-premises data center while continuing to maintain single source of truth datasets in AWS S3 in the “S” Region.
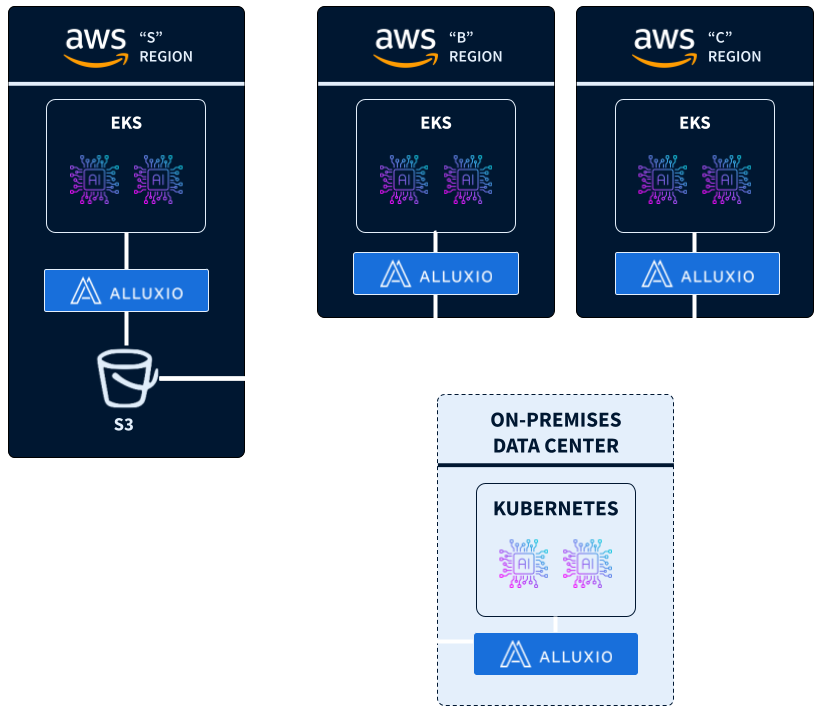
RESULTS
Since deploying Alluxio AI Enterprise, the company’s AI/ML training workloads have become faster and more stable, while also:
- Reducing AWS S3 API and egress charges by over 50%
- Improving GPU utilization by 20%
- Reducing operational complexity in their on-premises data center
.png)