Blog

We are thrilled to announce the general availability of Alluxio Enterprise for Data Analytics 3.2! With data volumes continuing to grow at exponential rates, data platform teams face challenges in maintaining query performance, managing infrastructure costs, and ensuring scalability. This latest version of Alluxio addresses these challenges head-on with groundbreaking improvements in scalability, performance, and cost-efficiency.
.jpeg)
We’re excited to introduce Rapid Alluxio Deployer (RAD) on AWS, which allows you to experience the performance benefits of Alluxio in less than 30 minutes. RAD is designed with a split-plane architecture, which ensures that your data remains secure within your AWS environment, giving you peace of mind while leveraging Alluxio’s capabilities.
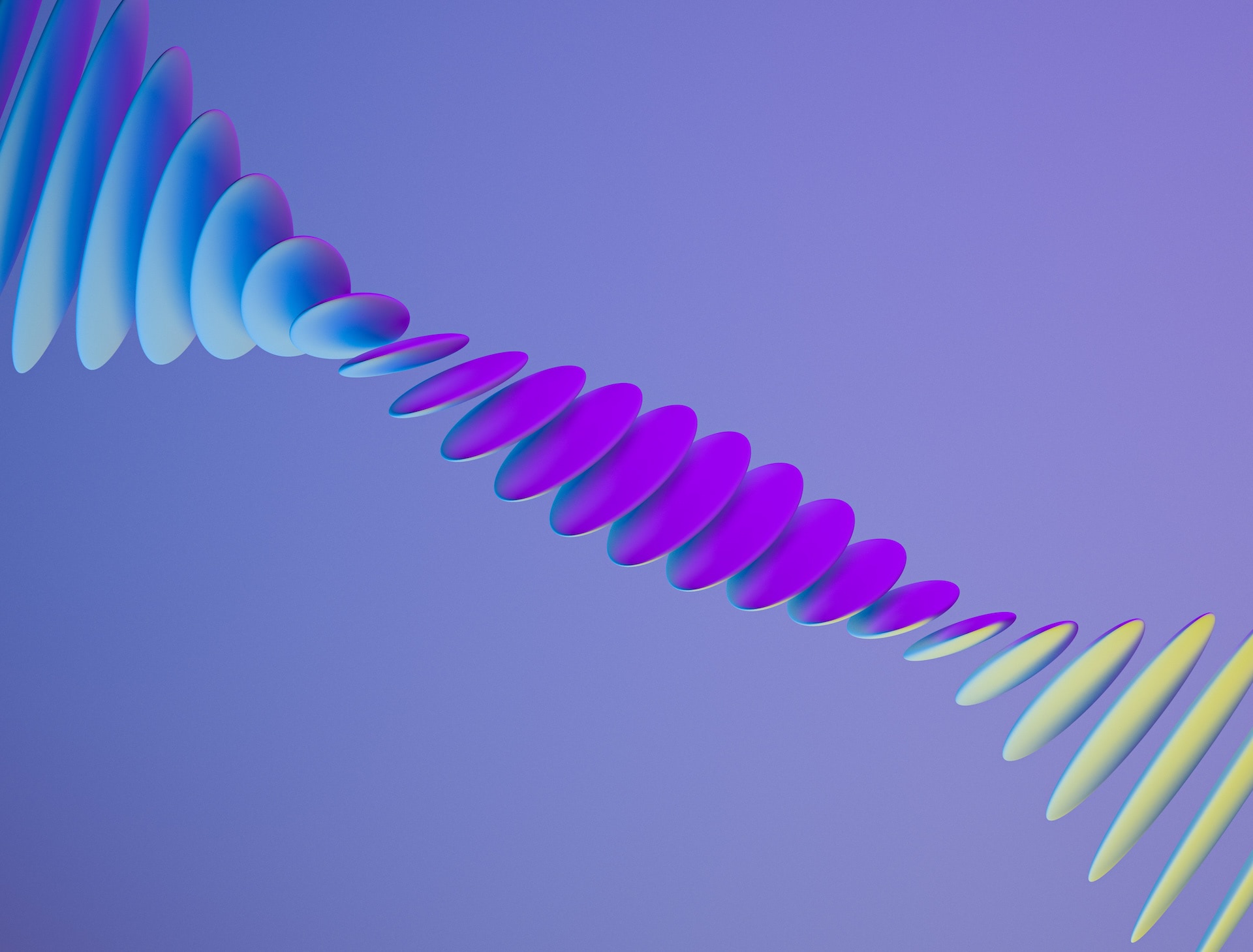
PyTorch is one of the most popular deep learning frameworks in production today. As models become increasingly complex and dataset sizes grow, optimizing model training performance becomes crucial to reduce training times and improve productivity.
.png)
.jpeg)
This article described how engineers at datasapiens brought down S3 API costs by 200x by implementing Alluxio as a data orchestration layer between S3 and Presto.
.jpeg)
As the third largest e-commerce site in China, Vipshop processes large amounts of data collected daily to generate targeted advertisements for its consumers. In this article, Gang Deng from Vipshop describes how to meet SLAs by improving struggling Spark jobs on HDFS by up to 30x, and optimize hot data access with Alluxio to create a reliable and stable computation pipeline for e-commerce targeted advertising.
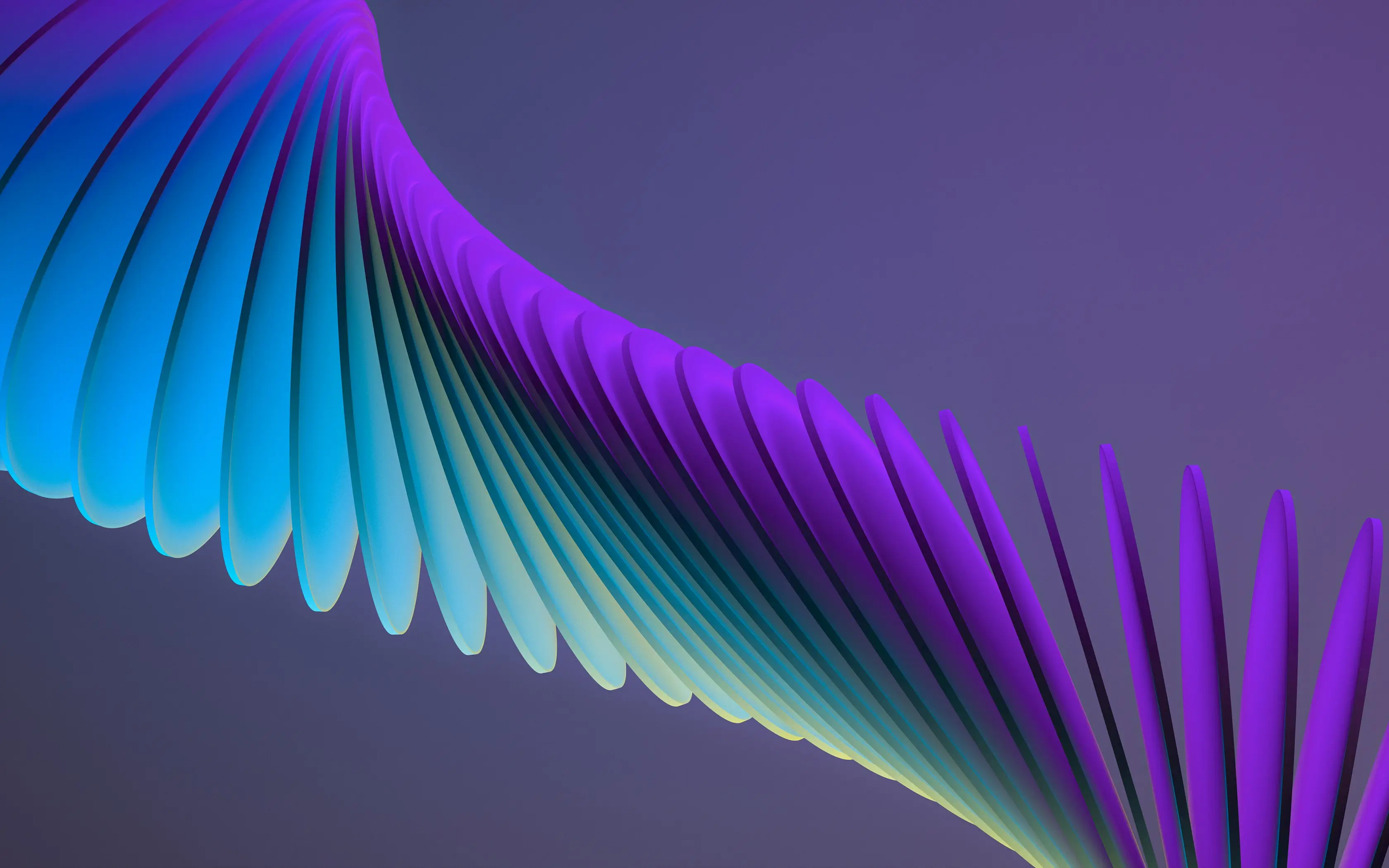
In this blog, Derek Tan, Executive Director of Infra & Simulation at WeRide, describes how engineers leverage Alluxio as a hybrid cloud data gateway for applications on-premises to access public cloud storage like AWS S3.
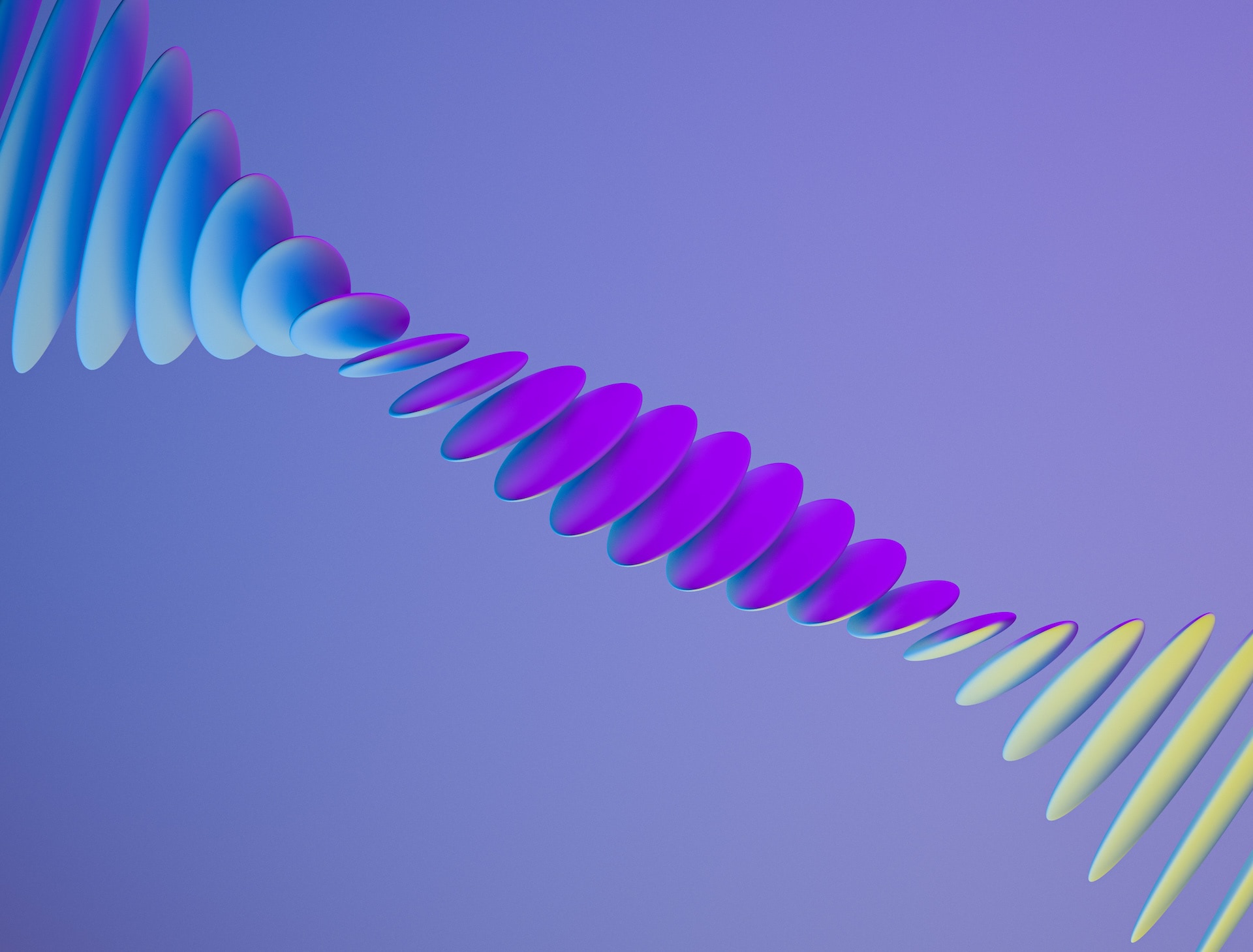
Alluxio 2.3.0 focuses on streamlining the user experience in hybrid cloud deployments where Alluxio is deployed with compute in the cloud to access data on-prem. Features such as environment validation tools and concurrent metadata synchronization greatly improve Alluxio’s functionality. Integrations with AWS EMR, Google Dataproc, K8s, and AWS Glue make Alluxio easy to use in a variety of cloud environments. In this article, we will share some of the highlights of the release. For more, please visit our release notes page.
.jpeg)
In this article, Honghan Tian describes how engineers in the Data Service Center (DSC) at Tencent PCG (Platform and Content Business Group) leverages Alluxio to optimize the analytics performance and minimize the operating costs in building Tencent Beacon Growing, a real-time data analytics platform.
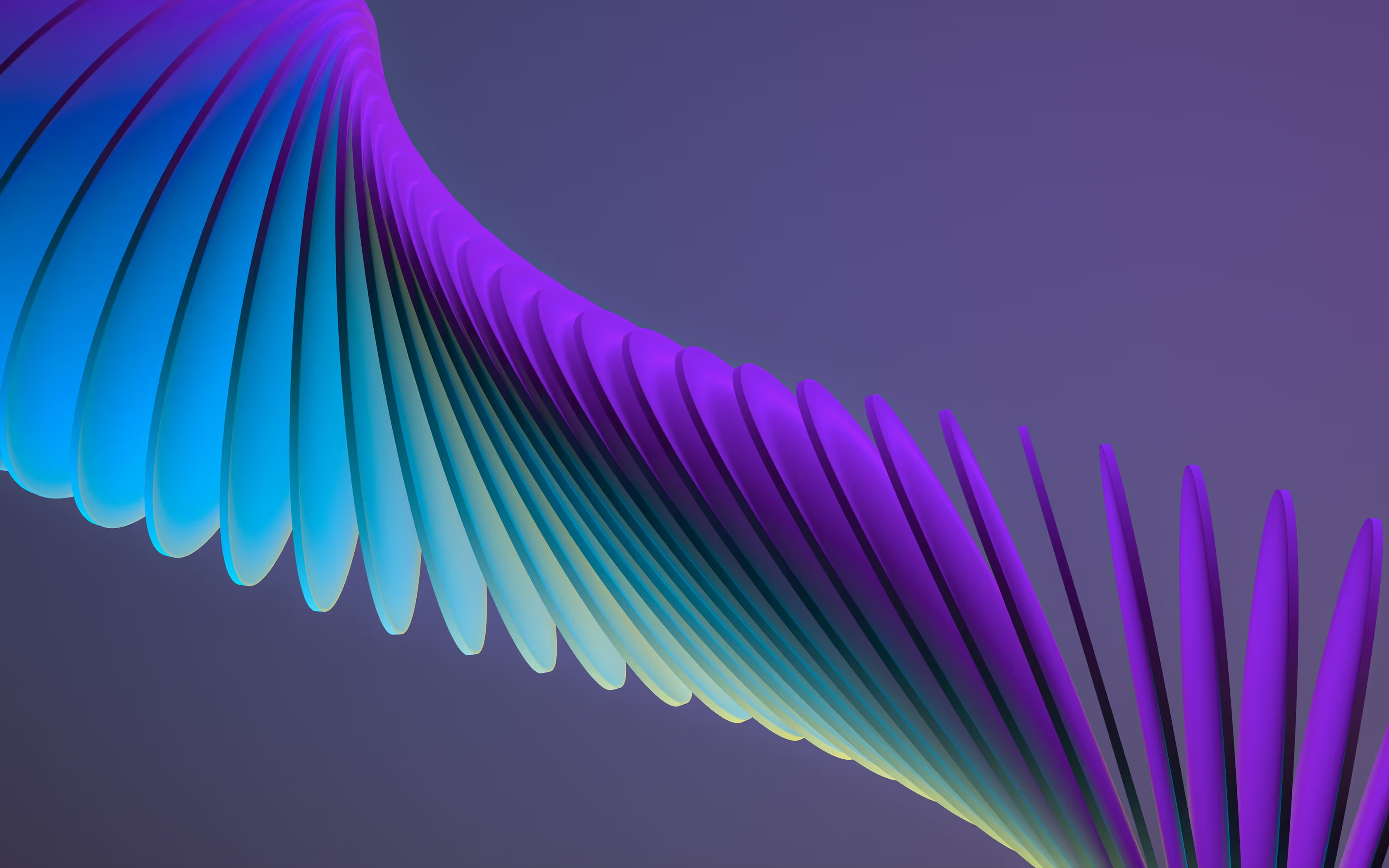
This article presents the collaboration of Alibaba, Alluxio, and Nanjing University in tackling the problem of Deep Learning model training in the cloud. Various performance bottlenecks are analyzed with detailed optimizations of each component in the architecture. Our goal was to reduce the cost and complexity of data access for Deep Learning training in a hybrid environment, which resulted in over 40% reduction in training time and cost.
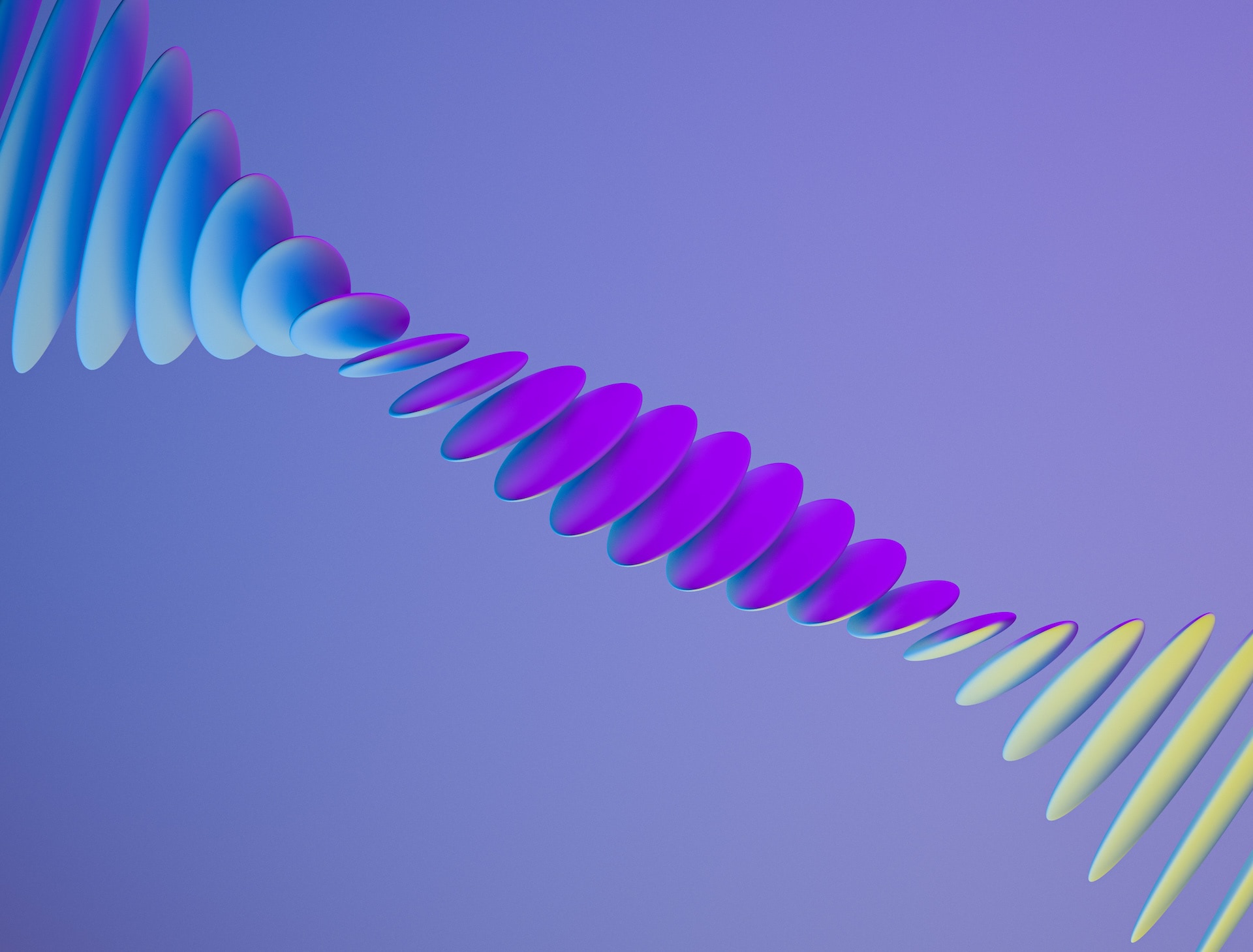
This article describes how Alluxio can accelerate the training of deep learning models in a hybrid cloud environment when using Intel’s Analytics Zoo open source platform, powered by oneAPI. Details on the new architecture and workflow, as well as Alluxio’s performance benefits and benchmarks results will be discussed.
.jpeg)
Are you using SQL engines, such as Presto, to query existing Hive data warehouse and experiencing challenges including overloaded Hive Metastore with slow and unpredictable access, unoptimized data formats and layouts such as too many small files, or lack of influence over the existing Hive system and other Hive applications?