Blog
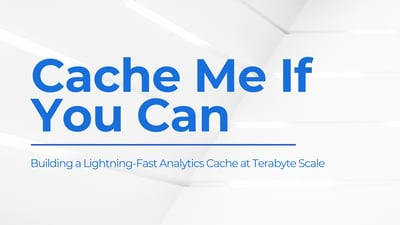
Suresh Kumar Veerapathiran and Anudeep Kumar, engineering leaders at Uptycs, recently shared their experience of evolving their data platform and analytics architecture to power analytics through a generative AI interface. In their post on Medium titled Cache Me If You Can: Building a Lightning-Fast Analytics Cache at Terabyte Scale, Veerapathiran and Kumar provide detailed insights into the challenges they faced (and how they solved them) scaling their analytics solution that collects and reports on terabytes of telemetry data per day as part of Uptycs Cloud-Native Application Protection Platform (CNAPP) solutions.
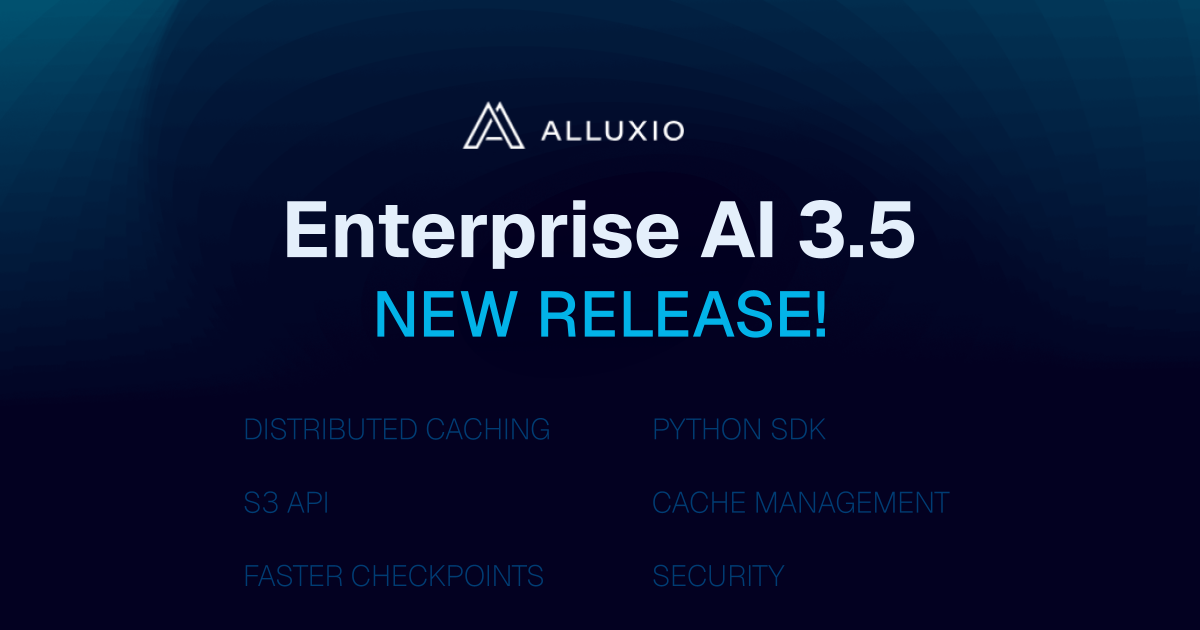
With the new year comes new features in Alluxio Enterprise AI! Just weeks into 2025 and we are already bringing you exciting new features to better manage, scale, and secure your AI data with Alluxio. From advanced cache management and improved write performance to our Python SDK and S3 API enhancements, our latest release of Alluxio Enterprise AI delivers more power and performance to your AI workloads. Without further ado, let’s dig into the details.
.png)

This is a blog series talking about the design and implementation of the Cross Cluster Synchronization mechanism in Alluxio. This mechanism ensures that the metadata is consistent when running multiple Alluxio clusters. Part 1 of this blog series discusses the scenario and background.
.jpeg)
.jpeg)
Modern analytics projects rely on a hodgepodge of compute clusters, data stores, and pipelines, flung across countries and continents. Enterprises struggle to meet performance SLAs without replicating lots of data or moving and re-coding applications.
.jpeg)
.jpeg)
The problem with data modernization initiatives is that they result in distributed datasets that impede analytics projects. As enterprises start their cloud migration journey, adopt new types of applications, data stores, and infrastructure, they still leave residual data in the original location. This results in far-flung silos that can be slow, complex and expensive to analyze. As business demands for analytics rise—along with cloud costs—enterprises need to rationalize how they access and process distributed data. They cannot afford to replicate entire datasets or rewrite software every time they study data in more than one location.