Blog

We are thrilled to announce the general availability of Alluxio Enterprise for Data Analytics 3.2! With data volumes continuing to grow at exponential rates, data platform teams face challenges in maintaining query performance, managing infrastructure costs, and ensuring scalability. This latest version of Alluxio addresses these challenges head-on with groundbreaking improvements in scalability, performance, and cost-efficiency.
.jpeg)
We’re excited to introduce Rapid Alluxio Deployer (RAD) on AWS, which allows you to experience the performance benefits of Alluxio in less than 30 minutes. RAD is designed with a split-plane architecture, which ensures that your data remains secure within your AWS environment, giving you peace of mind while leveraging Alluxio’s capabilities.
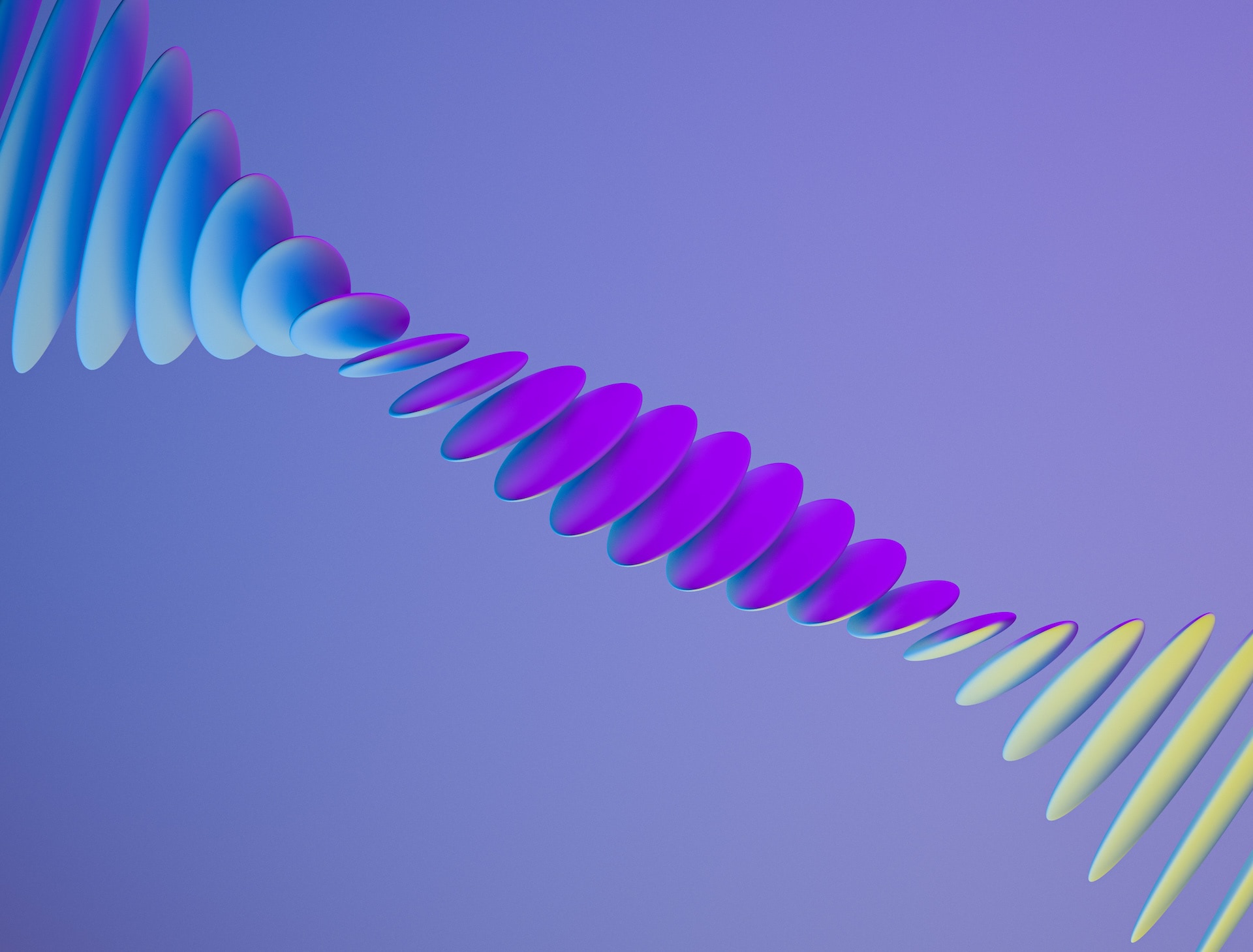
PyTorch is one of the most popular deep learning frameworks in production today. As models become increasingly complex and dataset sizes grow, optimizing model training performance becomes crucial to reduce training times and improve productivity.
.png)
.jpeg)
Alluxio is a new layer on top of under storage systems that can not only improve raw I/O performance but also enables applications flexible options to read, write and manage files. This article focuses on describing different ways to write files to Alluxio, realizing the tradeoffs in performance, consistency, and also the level of fault tolerance compared to HDFS.
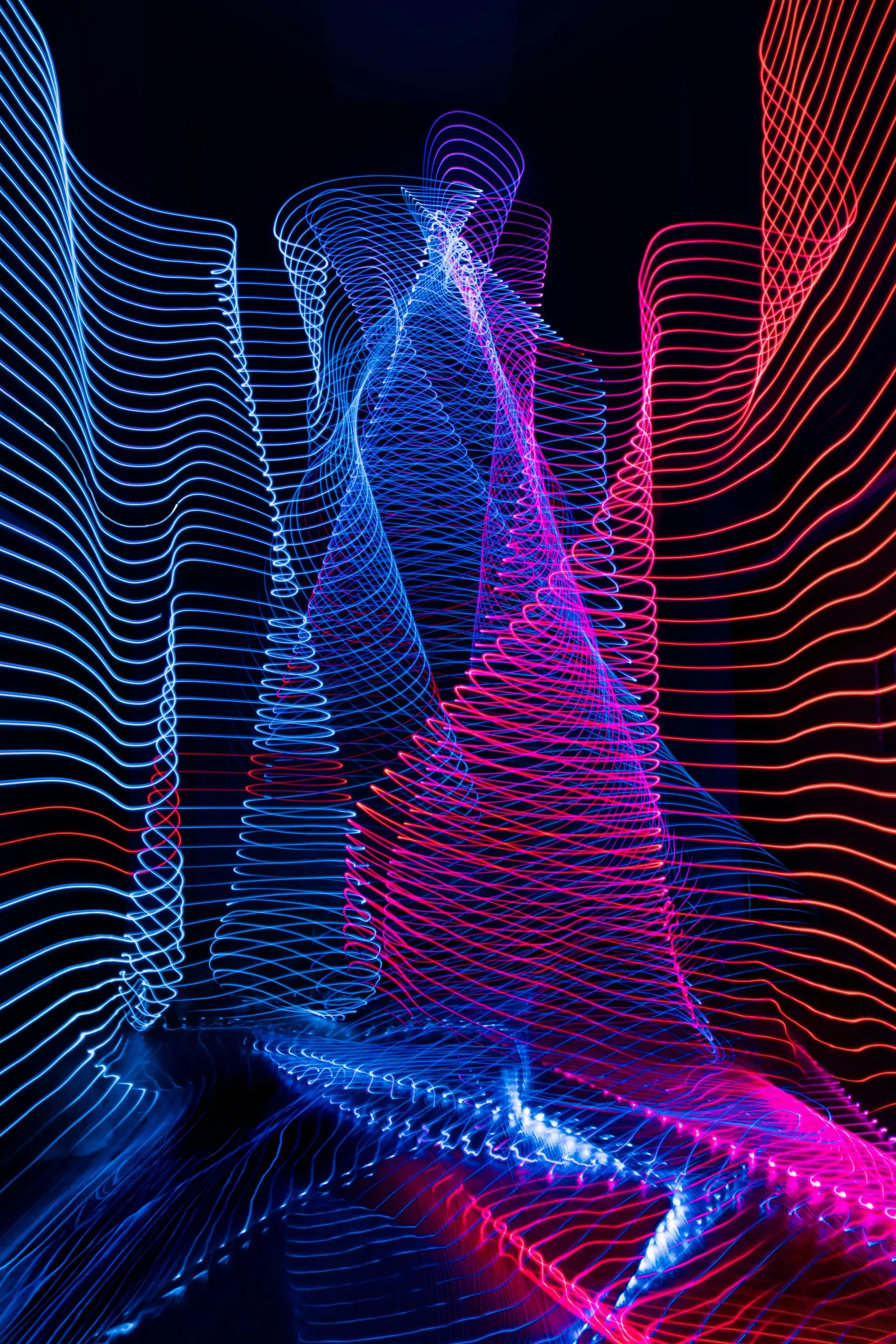
Monitoring metrics is highly important to operate distributed systems in production. Alluxio collects metrics using the Codahale Metrics Library on I/O throughput, RPC throughput, and resource usage. Alluxio metrics are shown in its webUI, but are also available through a REST endpoint or exportable to several third-party sinks in a time-series manner (see docs).
.jpeg)
Alluxio is an open-source data orchestration system widely used to speed up data-intensive workloads in the cloud. Alluxio v2.0 introduced Replicated Async Write to allow users to complete writes to Alluxio file system and return quickly with high application performance, while still providing users with peace of mind that data will be persisted to the chosen under storage like S3 in the background.
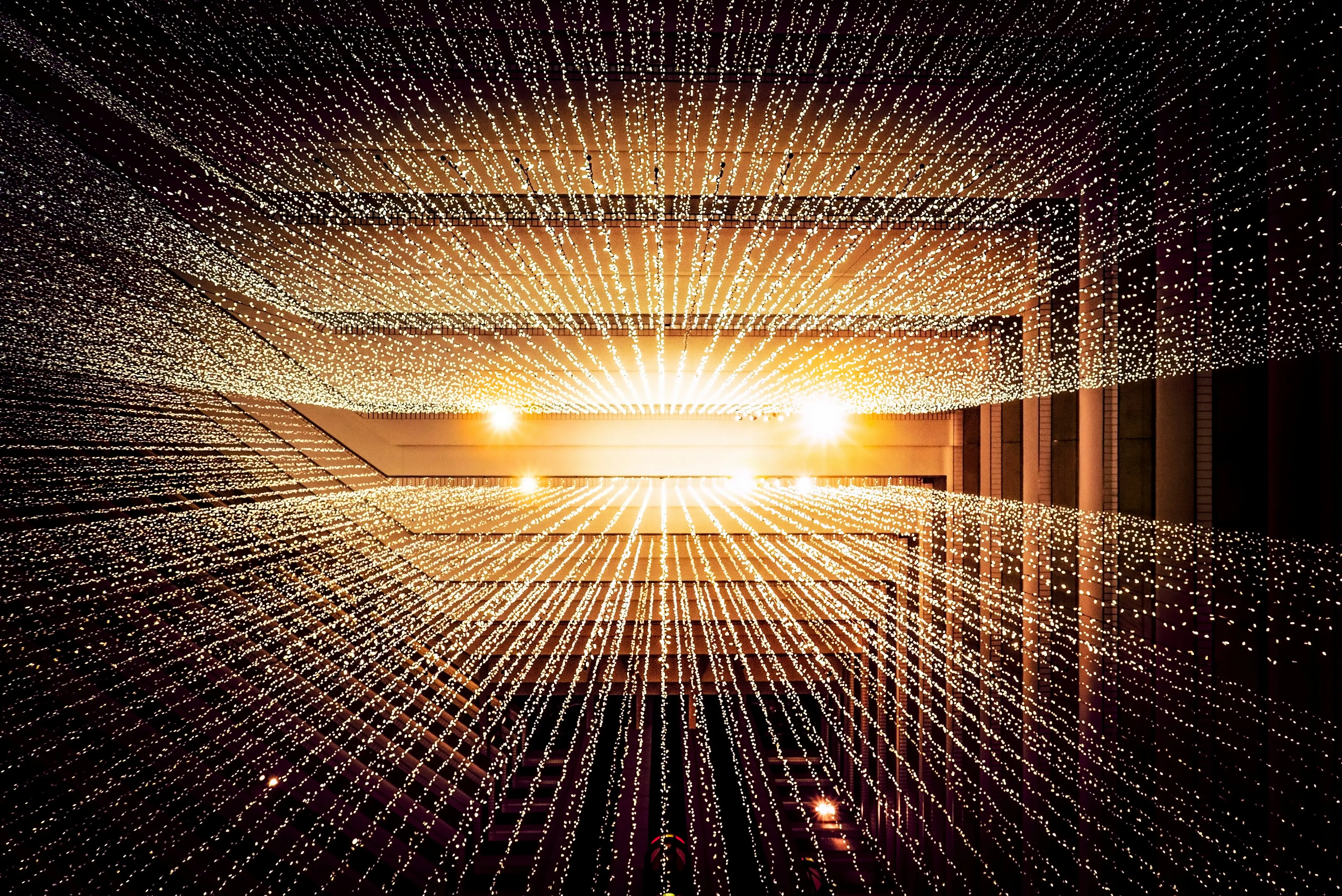
Alluxio is a proud sponsor and exhibitor at the AWS Summit in New York. If you weren't able to attend, here are the highlights
.jpeg)
Today, real-time computation platform is becoming increasingly important in many organizations. In this article, we will describe how ctrip.com applies Alluxio to accelerate the Spark SQL real-time jobs and maintain the jobs’ consistency during the downtime of our internal data lake (HDFS). In addition, we leverage Alluxio as a caching layer to dramatically reduce the workload pressure on our HDFS NameNode.
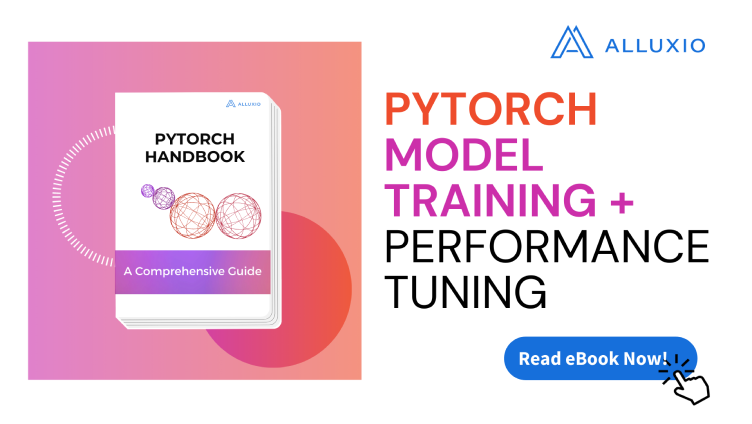
Today, I’m thrilled to announce the GA of Alluxio 2.0.0, Alluxio’s biggest release to date (see our Release Notes & Release Blog) with over 900 commits.
.jpeg)
Here in New York, at the AWS Summit, we are super excited to announce that Alluxio 2.0 is here, our most major release since the Alluxio launch. A couple months ago, we released 2.0 Preview - which included some of the capabilities, but 2.0 now includes even more, to continue building on to our data orchestration approach for the cloud.
.jpeg)
This article aims to provide a different approach to help connect and make distributed files systems like HDFS or cloud storage systems look like a local file system to data processing frameworks: the Alluxio POSIX API. To explain the approach better, we used the TensorFlow + Alluxio + AWS S3 stack as an example.
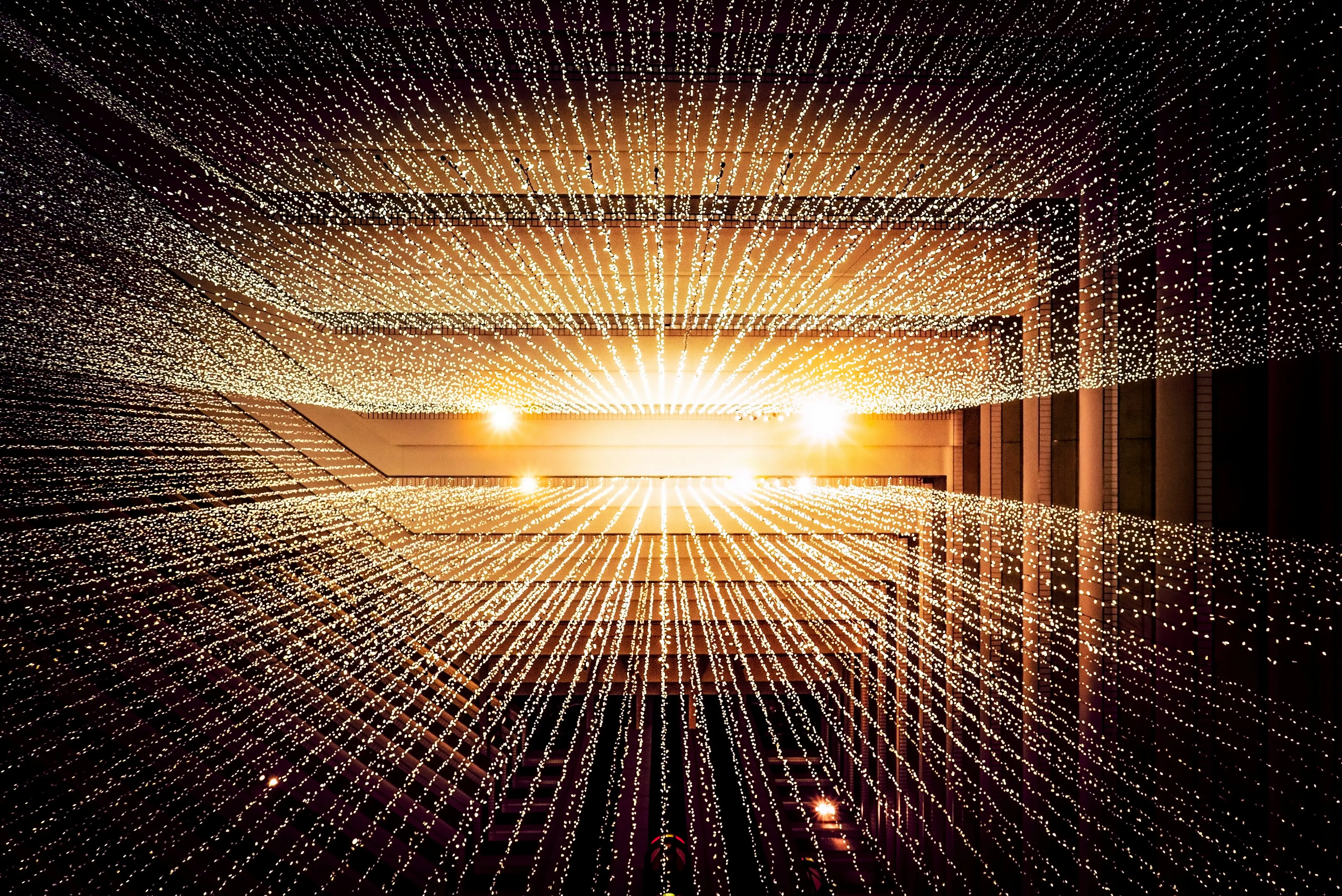
Alluxio is a proud sponsor and exhibitor at the Presto Summit in San Francisco. If you missed the conference, don’t worry we’ve got you covered!
.jpeg)
Cloud has changed the dynamics of data engineering as well as the behavior of data engineers in many ways. This is primarily because a data engineer on premise only dealt with databases and some parts of the hadoop stack. In the cloud, things are a bit different. Data engineers suddenly need to think different and broader. Instead of being purely focused on data infrastructure, you are now almost a full stack engineer (leaving out the final end application perhaps). Compute, containers, storage, data movement, performance, network — skills are increasing needed across the broader stack. Here are some design concept and data stack elements to keep in mind.
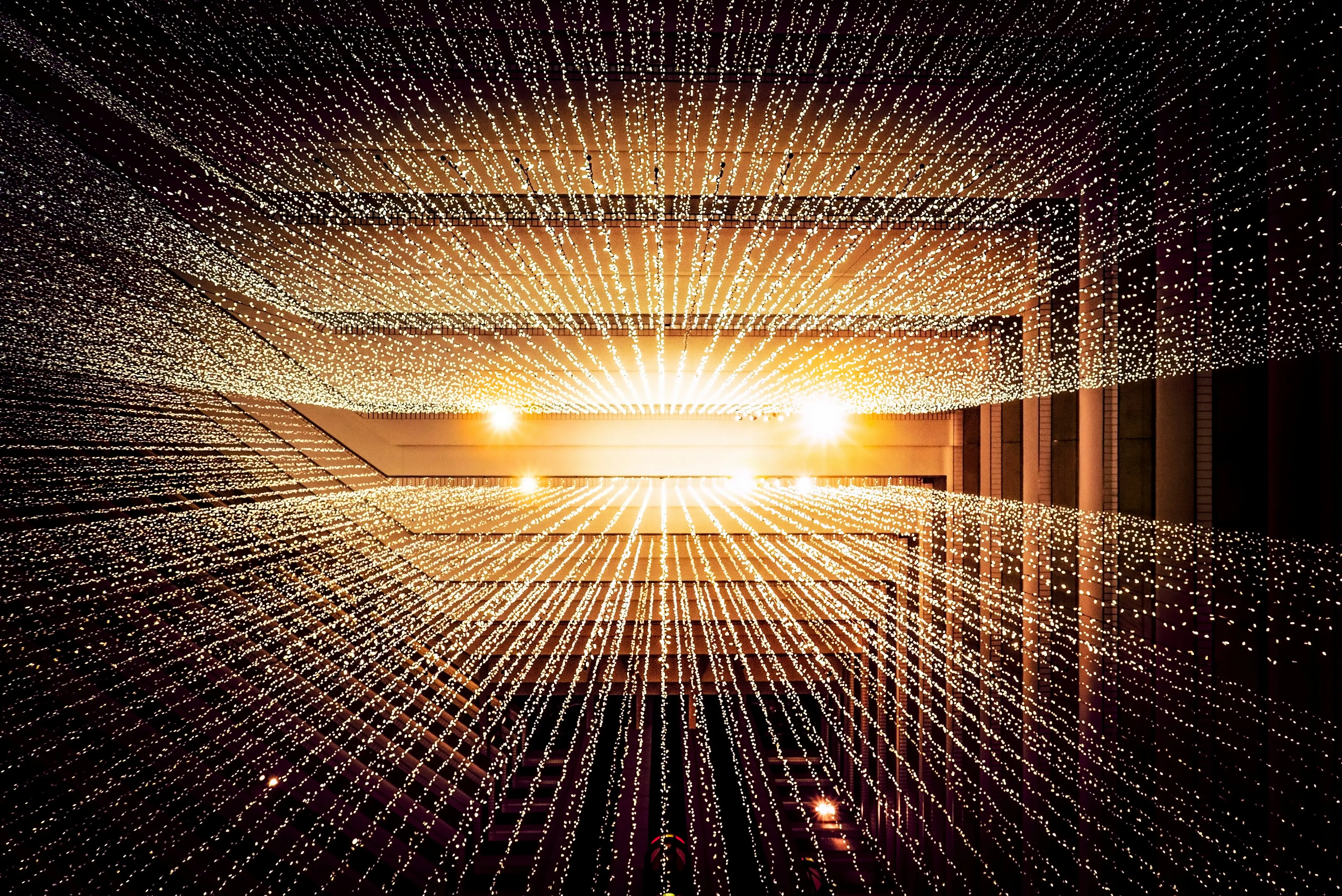
Over the years of working in the big data and machine learning space, we frequently hear from data engineers that the biggest obstacle to extracting value from data is being able to access the data efficiently. Data silos, isolated islands of data, are often viewed by data engineers as the key culprit or public enemy №1. There have been many attempts to do away with data silos, but those attempts themselves have resulted in yet another data silo, with data lakes being one such example. Rather than attempting to eliminate data silos, we believe the right approach is to embrace them.