Lenovo is an Alluxio customer with a common problem and use case in the world of data analytics. They have petabytes of data in multiple data centers in different geographic locations. Analyzing it requires an ETL process to get all of the data in the right place. This is both slow, because data has to be transferred across the network, and costly because multiple copies of the data need to be stored. Freshness and quality of the data can also suffer as the data is also potentially out of date and incomplete because regulatory issues prevent certain data from being transferred. Lenovo deployed Alluxio to address these issues and eliminate the need for ETL altogether. Data from multiple locations is cached in Alluxio and multiple applications can access it for analysis. The data is either accessed locally from memory or fetched by Alluxio for new requests as needed from persistent storage. The working data set is always available without the need for ETL. Alluxio fits within the existing security frameworks and enforces the policies in place, ensuring regulatory and compliance requirements from different countries and jurisdictions are met. You can learn more in the full case study
.png)
Blog
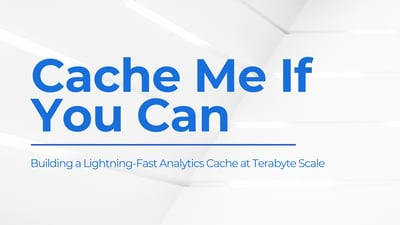
Suresh Kumar Veerapathiran and Anudeep Kumar, engineering leaders at Uptycs, recently shared their experience of evolving their data platform and analytics architecture to power analytics through a generative AI interface. In their post on Medium titled Cache Me If You Can: Building a Lightning-Fast Analytics Cache at Terabyte Scale, Veerapathiran and Kumar provide detailed insights into the challenges they faced (and how they solved them) scaling their analytics solution that collects and reports on terabytes of telemetry data per day as part of Uptycs Cloud-Native Application Protection Platform (CNAPP) solutions.
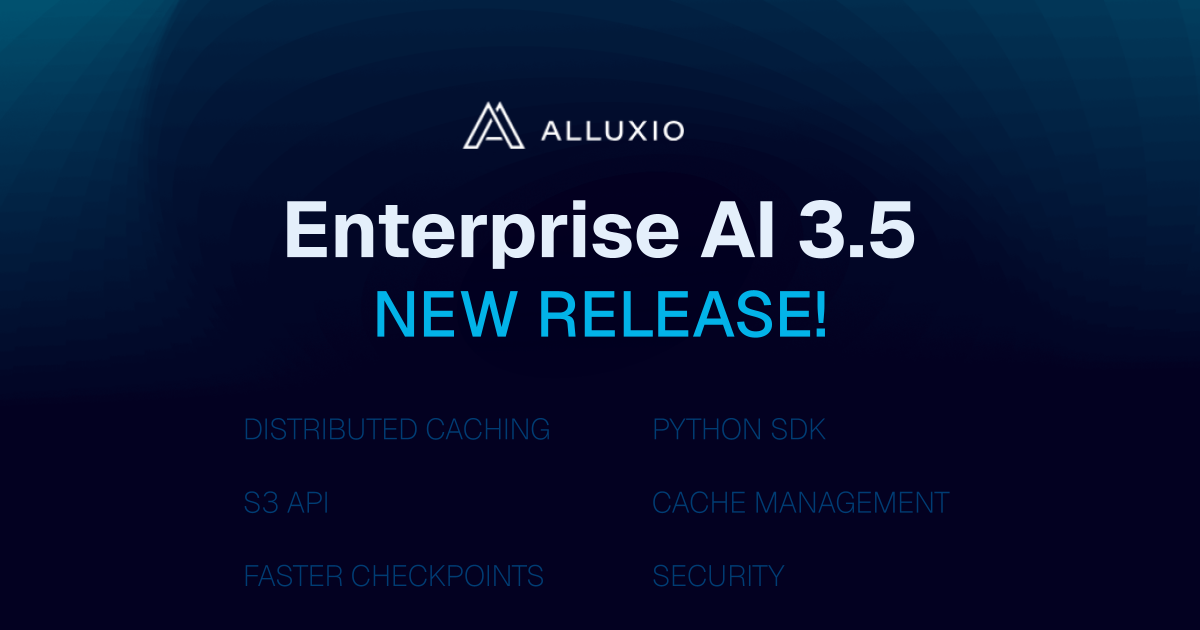
With the new year comes new features in Alluxio Enterprise AI! Just weeks into 2025 and we are already bringing you exciting new features to better manage, scale, and secure your AI data with Alluxio. From advanced cache management and improved write performance to our Python SDK and S3 API enhancements, our latest release of Alluxio Enterprise AI delivers more power and performance to your AI workloads. Without further ado, let’s dig into the details.