This article was initially posted on datanami.
The paradigm shift ushered in by Artificial Intelligence (AI) in today’s business and technological landscapes is nothing short of revolutionary. AI’s potential to transform traditional business models, optimize operations, and catalyze innovation is vast. But navigating its complexities can be daunting. Organizations must understand and adhere to some foundational principles to ensure AI initiatives lead to sustainable success. Let’s delve deeper into these ten evergreen principles:
1. Define Your Purpose
Every expedition begins with a map and destination in mind. Similarly, your AI journey should start with a vividly defined purpose. What are the specific challenges you seek to address using AI? Is it about improving customer engagement, streamlining supply chains, or uncovering new revenue streams? Establishing clear objectives upfront ensures that all subsequent efforts align with the broader business goals, facilitating a focused and purpose-driven AI strategy.
Examples:
- Implementing AI might enhance patient care by predicting disease outbreaks and optimizing resource allocation in real time for a healthcare provider.
- The goal of the retail industry is to use AI for personalized recommendations, thereby improving customer satisfaction and increasing sales.
2. Build Collaborative Relationships
The realm of AI is vast, with its intricacies evolving daily. No organization can claim to have all the answers. Therefore, partnerships with innovative startups, academic researchers, and industry stalwarts can be invaluable. Such collaborations bring fresh perspectives, diverse expertise, and shared learnings. By standing on the shoulders of giants and peers alike, businesses can leapfrog common pitfalls and accelerate their AI journey.
Examples:
- A manufacturing company partners with a robotics startup to integrate AI-driven automation into their production processes.
- An e-commerce platform collaborates with university data scientists to develop advanced recommendation algorithms.
3. Excel in Analytics
Data is the raw material that fuels AI. But data, in its raw form, is akin to unrefined gold. Through the crucible of analytics, this raw data transforms into actionable intelligence. Mastering analytics involves understanding data sources, refining collection methods, and employing sophisticated tools to decipher patterns. AI plays a pivotal role in business decisions, so having a stronghold on analytics becomes the linchpin for success.
Examples:
- A bank uses advanced analytics to detect unusual transaction patterns and potential fraud by analyzing customer transaction data in real-time.
- An automobile manufacturer employs analytics to optimize its production line, predicting equipment failures and scheduling maintenance proactively based on sensor data.
4. Prioritize Agility in Architecture
The technological landscape is dynamic. Solutions that are relevant today might become obsolete tomorrow. As such, building an agile IT infrastructure is paramount. This agility extends beyond just cloud adoption. It encompasses choosing the right platforms, ensuring interoperability, and maintaining flexibility to pivot. An agile architecture also reduces dependency on a single vendor, ensuring the organization’s needs always come first.
Examples:
- An agile IT infrastructure allows a financial institution to integrate new fraud detection models as fraud techniques evolve seamlessly.
- A media company quickly adapts to changing viewer preferences by having an agile architecture for content delivery across various platforms.
5. Integrate AI into Workflows
AI should augment human capabilities, not replace them. To this end, design AI solutions to fit naturally within organizational workflows. When AI tools and systems feel like natural extensions of current processes, they are more readily adopted and utilized to their full potential. This results in minimal disruptions and maximum gains in efficiency.
Examples:
- A call center integrates AI-powered chatbots to assist human agents in handling routine customer inquiries, allowing agents to focus on more complex issues.
- AI is integrated into the checkout process to recommend complementary products to customers, increasing cross-selling opportunities seamlessly.
6. Aim for Holistic Transformation
AI’s capabilities aren’t just for one department or another; its transformative potential is organization-wide. Whether it’s HR leveraging AI for talent acquisition, finance using AI for predictive analytics, or operations employing AI for optimization, every department stands to benefit. By taking a holistic view, organizations can realize comprehensive growth and innovation.
Examples:
- An insurance company applies AI across its departments for claims processing, underwriting, and customer support to create a more efficient and customer-centric operation.
- AI optimizes a logistics company’s routes, warehouse operations, and inventory management, improving overall supply chain efficiency.
7. Uphold Effective Governance
As with any powerful tool, AI needs guidelines and oversight. Effective governance involves establishing ethical guidelines, ensuring data privacy, monitoring AI’s decisions for biases, and aligning AI’s outputs with organizational values. Organizations build trust and foster responsible innovation by ensuring that AI’s deployment aligns with business objectives and ethical considerations.
Examples:
- A medical research institution implementing AI for disease diagnosis would ensure its AI algorithms are regularly audited to maintain accuracy and prevent potential biases in patient treatment recommendations.
- In law enforcement, AI-driven predictive policing systems would undergo strict oversight to ensure they do not disproportionately target specific communities and adhere to ethical guidelines.
8. Nurture Expertise
The intricacies of AI require specialized knowledge. Investing in talent — hiring experts and upskilling existing teams — ensures that AI strategies are implemented effectively and evolve with the changing landscape. Centers of excellence serve as hubs of knowledge, research, and best practices, ensuring that AI initiatives are always at the cutting edge.
Examples:
- A technology company may establish a center of excellence for AI, where data scientists and engineers collaborate on cutting-edge research and development projects.
- A healthcare organization could provide training programs to upskill its workforce in AI, ensuring that medical professionals can effectively use AI-powered diagnostic tools.
9. Commit to Ongoing Investment
The AI journey is not a sprint but a marathon. Continuous capital, talent, and time investments are essential as technology evolves. This ensures that the AI systems remain relevant, efficient, and aligned with the latest in the field. A genuine commitment to AI goes beyond initial adoption, emphasizing sustained enhancement and growth.
Examples:
- A software company continuously invests in AI R&D, regularly updating its virtual assistant with new features and capabilities to stay competitive.
- A retail chain allocates a budget for ongoing AI investments, allowing it to stay ahead by implementing the latest inventory optimization algorithms and customer analytics tools.
10. Promote Data Diversity
An AI system is only as good as the data it’s trained on. Ensuring this data is diverse, comprehensive, and high-quality is essential. This means sourcing data from varied points, vetting it for accuracy, and ensuring it represents a broad spectrum. Diverse data leads to more robust AI models, minimizing biases and maximizing applicability.
Examples:
- A social media platform ensures its AI algorithms consider diverse user demographics and content types to prevent algorithmic bias and create a more inclusive user experience.
- In autonomous driving, training AI models on data from various geographic locations and weather conditions helps enhance the safety and reliability of self-driving vehicles.
Conclusion: The Pillars of a Robust AI Strategy
AI’s transformative potential is undeniable. However, the path to AI success is laden with challenges. The ten principles outlined serve as pillars to guide organizations on this journey. Embracing them ensures that AI initiatives are grounded in strategy, ethics, and expertise. As the horizon of AI expands, and its role in organizational success becomes ever more pronounced, these foundational principles will remain relevant, guiding both AI novices and veterans towards sustainable success.
.png)
Blog
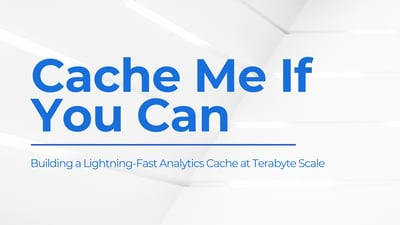
Suresh Kumar Veerapathiran and Anudeep Kumar, engineering leaders at Uptycs, recently shared their experience of evolving their data platform and analytics architecture to power analytics through a generative AI interface. In their post on Medium titled Cache Me If You Can: Building a Lightning-Fast Analytics Cache at Terabyte Scale, Veerapathiran and Kumar provide detailed insights into the challenges they faced (and how they solved them) scaling their analytics solution that collects and reports on terabytes of telemetry data per day as part of Uptycs Cloud-Native Application Protection Platform (CNAPP) solutions.
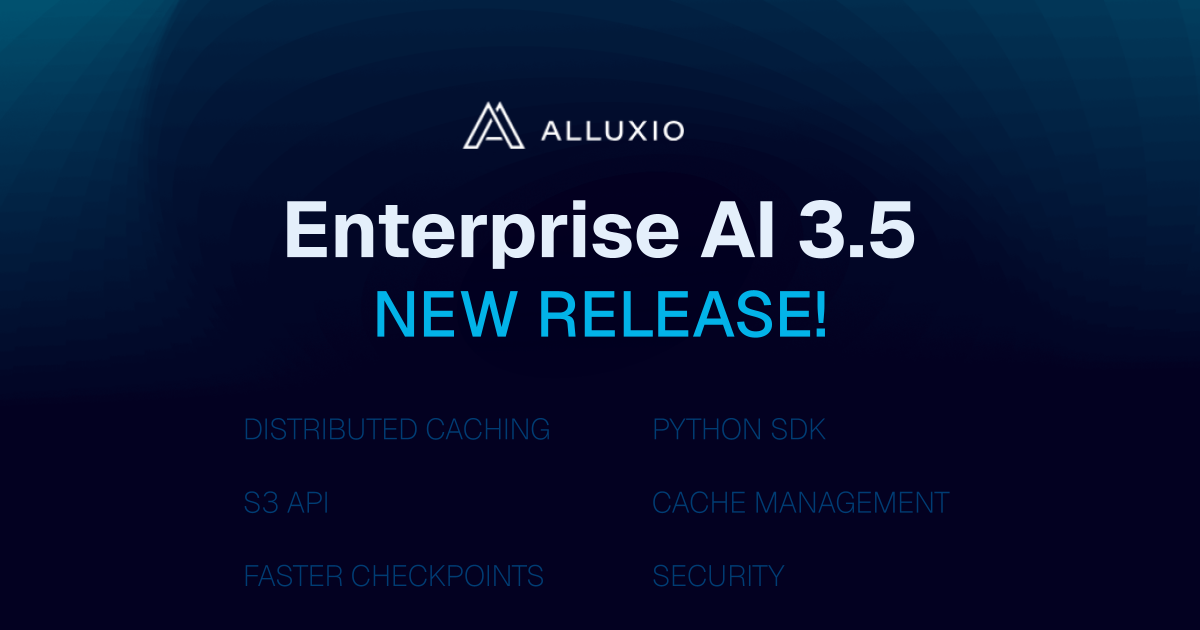
With the new year comes new features in Alluxio Enterprise AI! Just weeks into 2025 and we are already bringing you exciting new features to better manage, scale, and secure your AI data with Alluxio. From advanced cache management and improved write performance to our Python SDK and S3 API enhancements, our latest release of Alluxio Enterprise AI delivers more power and performance to your AI workloads. Without further ado, let’s dig into the details.